Alberto Testolin
Alberto Testolin
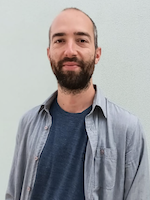
Assistant Professor
Department of General Psychology and Department of Mathematics, University of PadovaOffice: via Venezia 8, Psico 1 (6th floor)
35131 Padova (Italy)
e-mail: alberto.testolin(at)unipd.it
I am broadly interested in Artificial Intelligence, Cognitive Science and Computational Neuroscience. In the AI field, my research covers the theoretical and technological aspects of neural networks, with a particular focus on deep learning, large language models and the integration between statistical learning and symbolic reasoning. I also apply machine learning to signal processing, data science and system optimization. In the CS and CN fields, my interdisciplinary research approach focuses on the domains of visual perception, predictive coding, cognitive development, numerical cognition and mathematical learning using computer simulations based on artificial neural networks and brain models.
I am a member of the IEEE Task force on Deep Learning.
Education
Ph.D., Cognitive Science, University of Padova (2015)
Laurea (M.Sc.), Computer Science (Artificial Intelligence), University of Padova (2011)
Representative publications (see full list and PDF files here)
- Testolin, A., Kipnis, D., & Diamant, R. (2021). Detecting submerged objects using active acoustics and deep neural networks: A test case for pelagic fish. IEEE Transactions on Mobile Computing.
- Testolin A, Zou W, and McClelland, J (2020). Numerosity discrimination in deep neural networks: Initial competence, developmental refinement and experience statistics. Developmental Science.
- Testolin, A., Dolfi, S., Rochus, M., and Zorzi, M. (2020). Visual sense of number vs. sense of magnitude in humans and machines. Scientific Reports.
- Testolin A, Stoianov I, and Zorzi M (2017). Letter perception emerges from unsupervised deep learning and recycling of natural image features. Nature Human Behaviour.